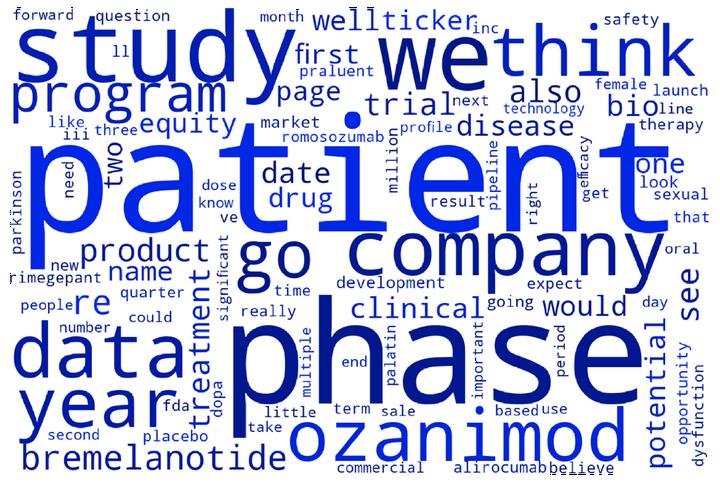
Accurately predicting the successful development of new pharmaceutical drugs is incredibly valuable for drug developers, investors, medical professionals, and patients. The standard approach is to use historical success rates stratified by basic features such as the product’s stage of development or disease area. Recently, researchers have turned to machine learning to incorporate additional features and employ more complex models.
A feature that has not yet been incorporated by previous research is the information disclosed during earnings calls and medical conferences by the company developing a given drug. Executives have access to non-public information related to a drug’s prospect of success (such as the results of a clinical trial that has not yet been published) and sometimes disclose this information for the first time in these meetings. Even when they do not disclose previously unknown information, they may indirectly communicate their optimism or pessimism though their word choice or sentiment.
In this paper we use natural language processing techniques to analyze information conveyed during earnings calls and conference presentations. We use word frequency, sentiment scores, and a set of basic drug features to classify which drugs succeeded or failed clinical development. Our dataset contains 3 groups of features: basic drug features (FDA designations, market cap, etc.), word frequencies, and sentiment scores. We employ 7 common machine learning algorithms.
Our results confirm the hypothesis that transcripts of earnings calls contain valuable information about whether a drug will succeed or fail. The performance of the models showed that the relative frequency of words used in these transcripts were reasonably predictive even in the absence of basic features of the drugs.